
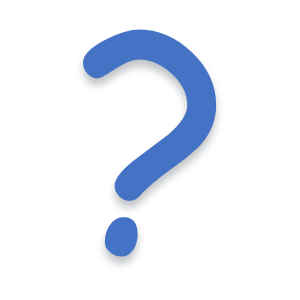
Its semantics, and a subject of ongoing debate.
Per wikipedia, I really like this proposal:
Astronomer Jean-Luc Margot proposed a mathematical criterion that determines whether an object can clear its orbit during the lifetime of its host star, based on the mass of the planet, its semimajor axis, and the mass of its host star.[210] The formula produces a value called π that is greater than 1 for planets.[c] The eight known planets and all known exoplanets have π values above 100, while Ceres, Pluto, and Eris have π values of 0.1, or less. Objects with π values of 1 or more are expected to be approximately spherical, so that objects that fulfill the orbital-zone clearance requirement around Sun-like stars will also fulfill the roundness requirement[211] – though this may not be the case around very low-mass stars.
It basically means a planet should be big enough to consolidate all the stuff in its orbital area, not be part of an asteroid field. That makes sense to me.
https://en.wikipedia.org/wiki/Dwarf_star
“Dwarf” stars are even more confusing, as it basically a synonym for “normal,” as opposed to “giant” stars (which are relatively puffy and big for their mass/temperature), or more exotic stars. But the term is also used for special cases, like the relatively exotic white dwarfs (remnants of exploded stars with very strange properties, extreme density, and not “burning” like a star traditionally does), or “barely a star” brown dwarfs.
TL:DR: If an astronomer asks you to name something, you should say ‘absolutely not.’
True, I suppose I though clearing the orbit sounded more colloquial, whereas gravitational dominance may not be as clear to some?